Outcomes
We will demonstrate and evaluate our novel and rigorous methods in a laboratory environment, on a range of use-cases. TUPLES will release some software tools and a testing environment to enable the human-centered development and assessment of trustworthy P&S systems.
Tuples Lab
To develop hybrid planning and scheduling methods that combine the efficiency, flexibility, and adaptability of data-driven learning approaches with the robustness, reliability, and clarity of model-based reasoning methods.
The TUPLES lab, will be a simulation environment enabling controlled experiments of trustworthy P&S methods on simplified versions of our use cases.
It will be an open access platform for experts and practitioners with tutorials, open access SW code and reusable libraries, customizable simulation environments for trustworthy hybrid P&S methodologies.
Using theTUPLES lab as a common development environment will improve interoperability of the new algorithmic techniques. The lab will also enable investigations that would be impossible on many of the original use cases. For example, one may be able to evaluate the robustness and safety of a system against adjustable degrees of uncertainty.
Similarly, the quality of the provided explanations may be assessed via experiments with recruited beta-testers (and supported by project staff with competence in humanities and social sciences).
During the project we also plan to rely on the TUPLES lab for running a research competition that will serve both to collect feedback on the environment, and for dissemination of the project results.
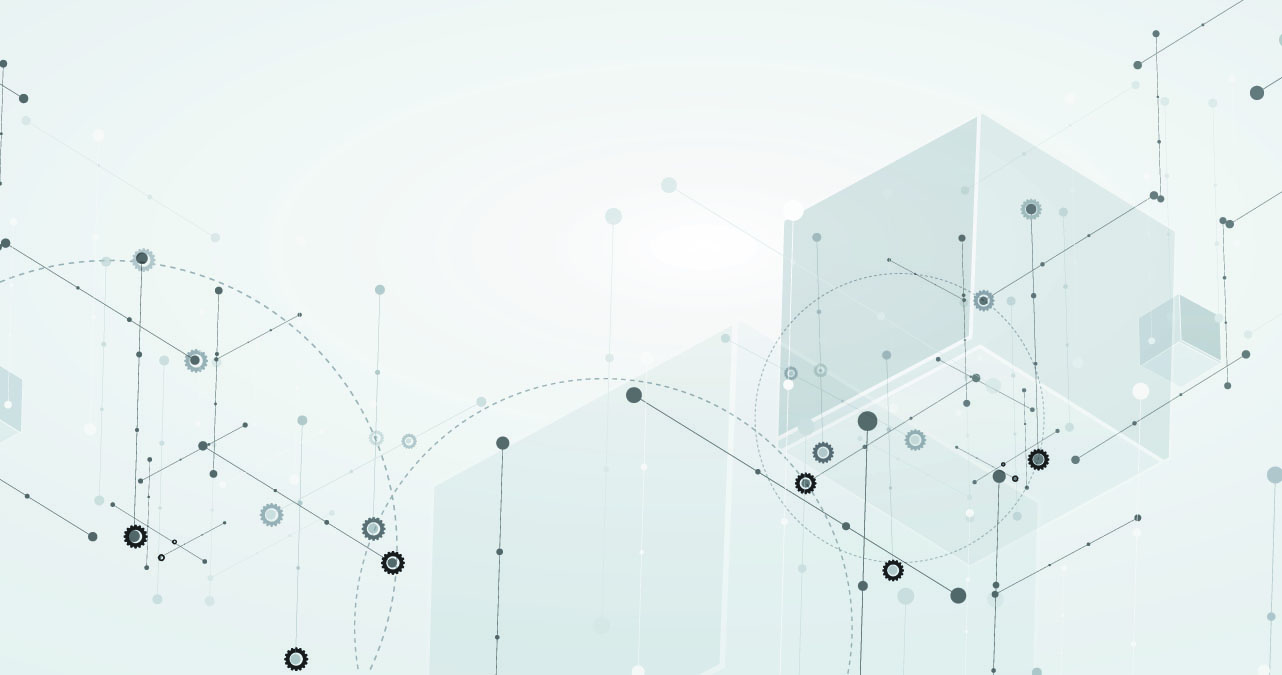
Self Assessment Tool
To develop verification and explanation methods capable of reasoning about the properties of the solutions produced by planning and scheduling systems, in particular when these are represented by neural networks.
The TUPLES self-assessment tool, an easy-to-use diagnostic survey, designed based on the experience matured in the course of the project, that any solution provider may adopt to evaluate the coherence of a specific method with respect to the key EU guidelines on trustworthy, reliable, robust and safe AI.
This tool will allow DSS developers to verify adherence to Trustworthy AI guidelines.
It’s first users are intended to be the EU business and academic community. The TUPLES self-assessment tool is going to be an independent tool that increases awareness of best practices and guidelines on Human-centered AI as well as assessing areas of potential improvement.
We will assess whether relevant metrics emerge that have the potential to evolve towards a real rating system that could eventually become a step towards a future certification standard.
Our hope is that this tool become the precursor of a possible future rating or standardisation scheme.
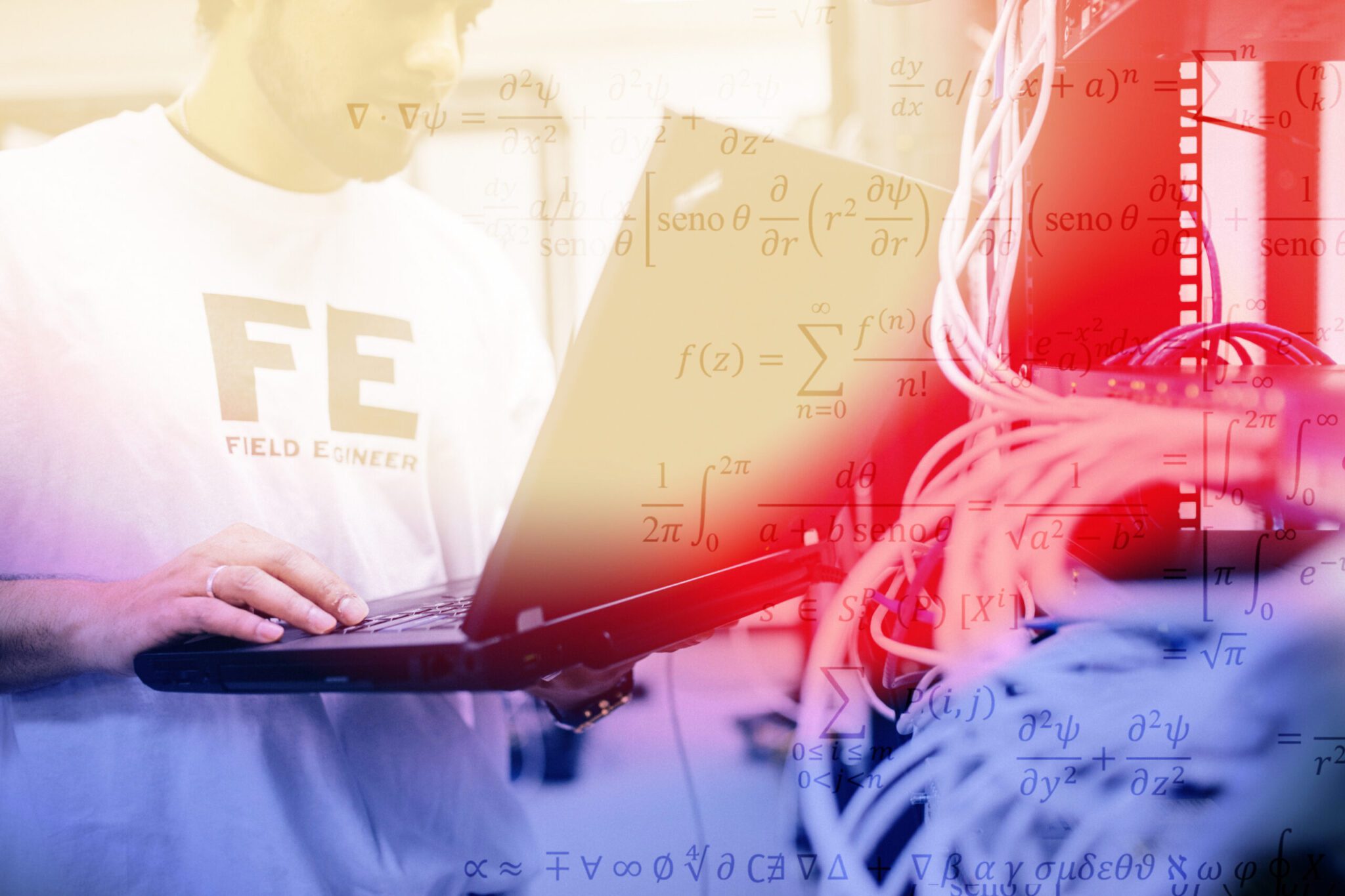
The TUPLES self-assessment tool, will be released in its beta version on september 2024 and in its definitive version at the end of the project (september 2025).
Impacts
To demonstrate these approaches on real practical case studies, from airplane pilot assistance, to soccer team recruitment, and waste collection.
The key and most important impact of the TUPLES project is the expected advance in Trustworthiness of AI, leading the way in research, development and deployment of world-class technologies that are beneficial to humans individually, organisationally and societally, adherent to European values, such as the principles reflected in our fundamental rights and environmental sustainability. To the extent AI developers produce tools that are (and are perceived as) safe, robust, explainable and scalable, they will be integrated in everyday practices of industries and citizens, spreading the benefits of increased performance and, more in general, enhanced decision making.
More specific examples of expected impacts are detailed for each use case in the appropriate section of the website.
Optimal and robust P&S processes will yield significant practical benefits, that can be classified across several areas:
AI-supported P&S will generally increase both efficiency and effectiveness of operations, reducing respectively the planning time and, most importantly, ensuring that models and algorithm capture the complexity of ever changing contextual conditions and recommend cost-optimal solutions, be they strategic design or operational management, supporting a general improvement in performance (which will ensure investment in AI is sustained by rapid returns).
Improved energy efficiency, reduced CO2 emissions and pollutants, lower dependence on fossil fuels and more efficient use of scarce (and costy) resources is the result of good planning in any resource- or energy-intensive process. The project includes a specific task to monitor and track environmental impacts across the 5 use cases, to provide real-life benchmarks of the potential of widespread application of AI-powered Best practices
The work environment will be positively impacted by the introduction of reliable and widely accepted P&S tools, increasing the competence, skills, and agency of human planners, foremen on the shop floor, and their workforce leading, for example, to more balanced shifts with reduced stress, limiting repetitive activities that can be performed by the AI (reducing cognitive load), and facilitating better management practices aided by explainable AI.